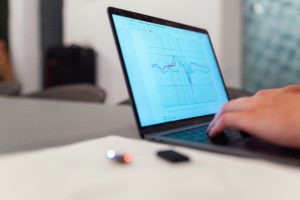
We live in a data-driven world, and as a data scientist and business turnaround advisor, we analyze a ton of data to uncover insights to help drive impactful solutions for our clients. We leveraged data analytics for finance forecasting as a critical means to help formulate effective turnaround plans and to support other high stakes business scenarios.
Data-driven analytics is a critical, evidenced-based strategy to validate business solutions, help gain buy-in from key stakeholders – and it can be the foundation of constructive negotiations. However, while numbers don’t (usually) lie, data alone doesn’t necessarily produce all the answers.
As the late George E. P. Box, a famous British great statistician once said, “All models are wrong, but some are useful.” There is truth in this statement, and we agree that data modeling isn’t the end-all be-all for predicting future outcomes. There is more involved.
You Need More Than Data Analytics for Finance Forecasting
In turnaround and restructuring situations, the stakes are high, and time is of the essence. You usually don’t have weeks to put together a plan and determine all the changes that need to be made. And you certainly don’t have the luxury to change course based on flawed assumptions. Therefore, in addition to analyzing the numbers, it’s imperative to evaluate the bigger picture, including historical drivers and performance elements. Most financial forecasts don’t factor in underlying drivers that may affect performance over time. Therefore, to be most useful, data modeling must also incorporate rational, defensible assumptions and capture the key drivers of the business.
When sticking with a simple income statement forecast, for example, some margin of error is okay “if and only if” you can communicate a realistic range of possible outcomes. An interpretation is simply not complete without additional considerations in restructuring consulting.
Effectively Translating Data Analytics
In financial forecasting, we’re not trying to be statisticians – we’re trying to be effective communicators. In addition to communicating our assumptions and translating expected performance, we must explain how we gathered and weighted this data and information. While there are many different approaches, a SWOT analysis is often a common tool used to assemble and supplement the data. Additionally, other approaches include prediction intervals, sensitivity analysis, multi-scenario bracketing, bootstrapping and bagging, and so on. And it depends on the sensitivities within each unique business. Only experience will tell you that. Like a life partner and the Five Languages of Love (great book), experience will guide you to the most effective form of communication.
CLIENT CASE STORY
A well-established retailer with brick-and-mortar stores (as well as e-commerce) was hit hard with the COVID-19 pandemic in 2020. Anxiety soared and leadership had to quickly determine the overall financial impact and how to effectively plan and manage through the situation. They engaged my team to help assess the situation and adjust to weather the COVID storm.
Approaching the Scenarios for the Best Solutions
In this scenario, we could have leveraged time-series machine learning with historical data to train the model and provide a quantified and properly qualified business prediction. However, it would have been incorrect because this approach assumed the future state was consistent with the historical state, which obviously wasn’t the case. We needed more than data. (And as far as I know, there isn’t any reliable data available from the 1918 Spanish Flu impact on e-commerce activity.)
Therefore, we used a data model with pre-pandemic activity to estimate what the expected activity should be as if there was no pandemic. Then, by comparing this data to real-time observations, we were able to quickly measure and identify pandemic impacts as they developed at different speeds in different markets. This information was extremely useful in proactively managing supply chain issues and leaning forward into upstream issues. None of this modeling was perfect but it was valuable in providing important data-driven insights in a timely manner.
In another scenario, this business continued to open new brick-and-mortar retail locations, expanding into new geographic markets. The conventional, intuitive wisdom was that it took generally 18 months post-market penetration to achieve a normalized, scaled volume of activity. In one metro area, there was uncertainty and debate about how the new locations were performing along this expected ramp up. Given that the market growth for a newly penetrated market was not expected to be linear on top of a business that had a complex, multi-phase seasonality, it was difficult for the leadership to have a reliable data-based assessment of performance. For an assessment, I was able to pull a wide sample of historical sales data for relatively similar situations, which allowed us to quantify a range of expected performance. We then normalized this projection for the complex seasonality with a modified Seasonally Adjusted Annual Rate conversion. The cliff-note version of the analysis demonstrated that this new market entrance was performing ~30% below where it should have been. Now of course, that 30% delta was ‘wrong’. There are other factors that could influence that behavior (e.g., supply chain disruptions, weather patterns, staffing issues, etc.) that wouldn’t have been quantified in the baseline model. But more importantly, the benefit in this analysis is that this ‘red-flag’ realization, by using strong analytics, was achieved well before the typical ‘wait and see’ approach played out. It provided enough predictive insight to allow the leadership team to find and focus on root cause issues and emplace countermeasures to maximize the value creation cycle.
Conclusion: Let data be a driver, but don’t forget the other passengers.
Leveraging fact-based data analytics is a critical strategy to drive future business decisions. However, data can’t stand alone and should be supplemented with other key factors and business drivers in order to be a useful tool.